
It is easiest to deduplicate rows in the primary data source when you have a dimension with unique Row IDs for each row in the data source. Unfortunately, after joining the Returns table to the Orders table, we get the inflated answer of $2,901,677. Let’s say we wanted to use the Sales measure from the primary data source the unjoined SUM of Sales is $2,297,201. The method described to this point is useful any time you are wanting to use measures from the secondary data source, but you can do something similar to ensure you are always using deduplicated values in the primary data source. This means that there was at least one item returned in 296 distinct orders.Īn Introduction to Tableau Level of Detail Expressions We’ve already aggregated the number of returns per Order ID with the MIN aggregation in the calculated field, but now I can also SUM up those number of returns. Level of detail expressions are unique in Tableau in that you can use an aggregate of an aggregate. If you use an aggregation of MIN, you will get one tally per Order ID. If I want to only count one return per order, I need to deduplicate the Yes tallies at the Order ID level of detail.
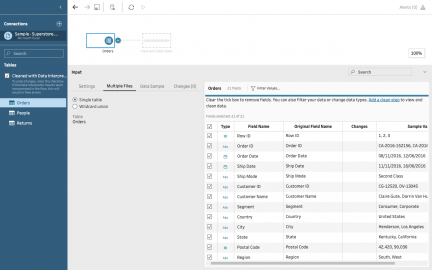
I know this number is inflated because the entire Returns tab in the Sample – Superstore Excel file only contains 800 rows. Placing this calculation on the Text Marks Card reveals the inflated answer of 3,226. Let’s say I want to count the number of returned orders and have created this calculated field to tally the number one every time the Returned dimension equals “Yes”. This becomes problematic when we try to use this joined dataset in a real-life scenario. This means I have 2,426 duplicate records.
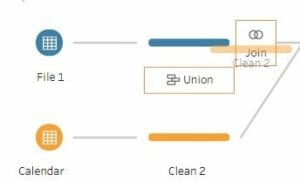
However, if I do the same check for the joined dataset, I get 12,420 records. unjoined) Orders table, this would result in 9,994 records. You can do this by simply placing the generated field called Number of Records onto the Text Marks Card.
#Tableau prep join how to#
First, to illustrate how to identify an issue, we’ll cover one of the first things I do when working with a new data source in Tableau, which is to view the number of records. The easiest way for Tableau authors to correct this type of row duplication is to create a calculated field that uses a FIXED LOD expression to isolate one value for the most granular level of detail in the join. How to deduplicate joined rows using Tableau’s Level of Detail expressions This can be very problematic for an untrained analyst because sales for the Newell 341 product in this order just went from $9 to $27. For example, Order ID CA-2015-143336 contains three products, and the join causes three rows (two of them new) to be created for each product. When this is the case, the join causes duplicate rows. The problem is that the Returns table only tells me if an order was returned at the Order ID level while the Orders table can have multiple rows per Order ID (in the case that multiple products were purchased in a single order). I could use this new column as a flag and/or create a calculated field to count the number of returns. For each Order ID, I will have a Yes or NULL to tell me which orders were returned. Since both tables contain the Order ID dimension, left joining the Returns table to the Orders table will add the new “Returned” column to the dataset. The join would look like this in Tableau Desktop:

For example, let’s say that I want to add a column from the Returns table to the Orders table of the Sample – Superstore dataset. My favorite way to use joins in Tableau is to add additional fields to my analysis when my primary table and the table containing the new fields have at least one dimension in common. The challenge of working with joined data sources in Tableau This post shares the challenge with joining multiple data sources and several solutions to ensure you are getting accurate answers – even when joining on multiple dimensions (i.e. Joins in Tableau are a powerful way to add new dimensions and measures to your analysis, but without a good understanding of how they affect your dataset, you will often end up with inflated numbers. They worked for a global company and needed to join a dataset containing monthly exchange rates to their primary data source. In one such case, an attendee was trying to solve the business problem of currency conversion. There are always problem-solving brainstorms at our Tableau training events, and sometimes the solutions are so relevant for all Tableau users that I want to share them on a larger scale.
